Imagine a world where understanding customer preferences is effortless, supply chains run flawlessly, and risks are identified before they materialize. This isn't science fiction; it's the reality powered by big data. From the seemingly endless streams of online activity to the intricate transactions within global corporations, vast amounts of data are generated every second. Harnessing this data effectively, however, requires sophisticated analytical tools and a keen understanding of its potential.
This exploration delves into the transformative power of big data, revealing how it's reshaping industries and driving unprecedented innovation.
Big data, characterized by its volume, velocity, variety, veracity, and value (the five Vs), offers businesses unprecedented insights. Analyzing this data allows for more accurate predictions, improved decision-making, and the creation of personalized customer experiences. We will examine how companies are using big data to optimize operations, reduce costs, develop innovative products, and mitigate risks across various sectors, from healthcare and finance to retail and manufacturing.
The journey ahead will reveal not only the benefits but also the challenges and the exciting future of this transformative technology.
Defining Big Data
Big data isn't just a lot of data; it's a paradigm shift in how we collect, analyze, and utilize information. It represents a massive volume of data that's too large or complex to be processed using traditional data processing applications. Understanding its characteristics and applications is crucial to harnessing its transformative power.The sheer scale and complexity of big data are defined by five key characteristics, often remembered by the acronym "5 Vs": Volume, Velocity, Variety, Veracity, and Value.
The Five Vs of Big Data
These five dimensions describe the challenges and opportunities presented by big data. Understanding them allows us to tailor our strategies for effective data management and analysis.
- Volume: This refers to the sheer quantity of data generated. We're talking terabytes, petabytes, and even exabytes of data – numbers that dwarf the capacity of traditional databases. Imagine the daily data generated by social media platforms, online transactions, or scientific research projects.
- Velocity: This signifies the speed at which data is generated and processed. In today's digital world, data streams in at an unprecedented rate, requiring real-time or near real-time processing capabilities. Think of stock market data, sensor readings from IoT devices, or live streaming video.
- Variety: Big data comes in many formats – structured, semi-structured, and unstructured. This diversity presents challenges for data integration and analysis but also unlocks richer insights. Examples include numerical data from databases, text from social media posts, and images from security cameras.
- Veracity: This relates to the trustworthiness and accuracy of the data. Big data sets often contain inconsistencies, errors, and biases, which must be addressed to ensure reliable analysis. Cleaning and validating data is crucial for obtaining meaningful results.
- Value: Ultimately, the value of big data lies in its ability to provide actionable insights. Extracting value requires effective data management, analysis, and visualization techniques. This value can manifest as improved decision-making, enhanced efficiency, and the creation of new products and services.
Types of Big Data
The diverse nature of big data necessitates different approaches to storage and processing. Understanding these different types is essential for effective data management.
- Structured Data: This is highly organized data that resides in a predefined format, typically relational databases. Examples include customer information in a CRM system or financial transactions in a banking database. This data is easily searchable and analyzable using traditional methods.
- Semi-structured Data: This data doesn't conform to a rigid table-like structure but still has some organizational properties. Examples include JSON and XML files, log files, and email messages. It's more flexible than structured data but requires specific tools for processing.
- Unstructured Data: This is the most challenging type to manage, lacking a predefined format. It includes text documents, images, audio files, and video recordings. Analyzing this data requires advanced techniques like natural language processing and image recognition.
Real-World Applications of Big Data
The impact of big data is pervasive, transforming industries and shaping our lives in countless ways.
- Personalized Recommendations: Companies like Netflix and Amazon use big data to analyze user preferences and provide personalized recommendations for movies, products, and services. This improves user experience and increases sales.
- Fraud Detection: Financial institutions utilize big data to identify fraudulent transactions in real-time. By analyzing patterns and anomalies, they can prevent losses and protect customers.
- Predictive Maintenance: Manufacturing companies use sensor data from machines to predict equipment failures and schedule maintenance proactively. This minimizes downtime and improves efficiency.
- Healthcare Improvements: Big data is used to analyze patient records, identify disease outbreaks, and develop personalized treatment plans. This leads to better patient outcomes and more efficient healthcare delivery.
- Traffic Optimization: Smart cities use big data from various sources (traffic cameras, GPS data, social media) to optimize traffic flow, reduce congestion, and improve transportation efficiency.
Benefits of Big Data in Business
Harnessing the power of big data is no longer a luxury; it's a necessity for businesses aiming to thrive in today's competitive landscape. The sheer volume, velocity, and variety of data available offer unprecedented opportunities for informed decision-making, enhanced customer relationships, and streamlined operations. This section explores the transformative impact of big data across key business functions.
Improved Decision-Making Processes
Big data analytics empowers businesses to move beyond gut feelings and anecdotal evidence, replacing them with data-driven insights. By analyzing vast datasets encompassing customer behavior, market trends, and internal operations, companies can identify patterns and predict future outcomes with greater accuracy. This allows for more strategic planning, resource allocation, and risk mitigation. For example, a retail company might analyze sales data to predict seasonal demand, optimizing inventory levels and avoiding stockouts or overstocking.
Predictive modeling, a cornerstone of big data analytics, allows businesses to anticipate customer needs and proactively address potential issues, ultimately leading to more effective and profitable decision-making.
Big Data's Impact on Customer Relationship Management (CRM)
Big data revolutionizes CRM by providing a 360-degree view of each customer. By integrating data from various sources – website interactions, social media activity, purchase history, customer service interactions – businesses can create detailed customer profiles. This granular understanding allows for personalized marketing campaigns, targeted product recommendations, and proactive customer service. For instance, an e-commerce platform can use big data to identify customers who are likely to churn and offer them targeted promotions or personalized support to retain their business.
This level of personalization fosters stronger customer loyalty and enhances the overall customer experience.
Big Data in Optimizing Supply Chain Management
Optimizing the supply chain is crucial for maintaining profitability and competitiveness. Big data analytics provides real-time visibility into every stage of the supply chain, from procurement to delivery. By analyzing data on inventory levels, transportation routes, and supplier performance, businesses can identify bottlenecks, predict disruptions, and optimize logistics. For example, a manufacturing company can use big data to predict potential delays in raw material delivery and proactively adjust its production schedule to minimize disruptions.
This proactive approach leads to improved efficiency, reduced costs, and enhanced customer satisfaction.
Comparison of Traditional Business Intelligence and Big Data Analytics
Feature | Traditional Business Intelligence | Big Data Analytics |
---|---|---|
Data Volume | Relatively small, structured data | Massive, varied, structured and unstructured data |
Data Sources | Limited to internal databases | Internal and external sources, including social media, IoT devices |
Analytical Techniques | Primarily reporting and dashboards | Advanced analytics, machine learning, predictive modeling |
Processing Speed | Relatively slow processing | Real-time or near real-time processing |
Big Data and Improved Operational Efficiency
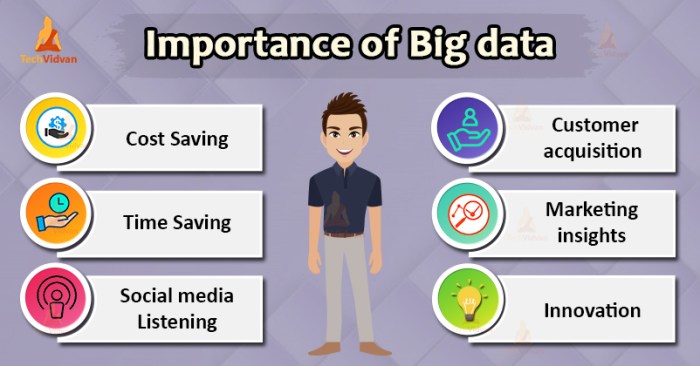
Big data's transformative power extends far beyond simple data analysis; it fundamentally reshapes operational efficiency across diverse industries. By leveraging the insights gleaned from massive datasets, organizations can streamline processes, optimize resource allocation, and ultimately, significantly reduce costs. This enhanced efficiency translates directly into increased profitability and a stronger competitive edge.Big data achieves this through sophisticated predictive modeling, real-time monitoring, and automated decision-making.
These capabilities allow businesses to anticipate potential problems, proactively address inefficiencies, and optimize performance in ways previously unimaginable. The result is a more agile, responsive, and cost-effective organization.
Predictive Maintenance and Reduced Downtime
Predictive maintenance, powered by big data analytics, revolutionizes how organizations manage their assets. By analyzing sensor data from machinery, equipment, or infrastructure, predictive models can forecast potential failures before they occur. This allows for proactive maintenance, minimizing downtime and extending the lifespan of assets. For example, a manufacturing plant might use big data to predict when a specific machine is likely to fail, scheduling maintenance during off-peak hours to avoid production disruptions.
This prevents costly emergency repairs and ensures continuous operation.
Optimized Supply Chain Management
Big data provides unparalleled visibility into the complexities of supply chains. Analyzing data on inventory levels, transportation routes, and customer demand allows businesses to optimize logistics, reduce waste, and improve delivery times. For instance, a retail company can use big data to predict future demand based on historical sales data and external factors like weather patterns. This allows them to optimize inventory levels, reducing storage costs and minimizing stockouts.
Real-time tracking of shipments allows for proactive responses to delays or disruptions, ensuring timely delivery of goods.
Improved Customer Service and Reduced Costs
Analyzing customer interaction data, including call logs, social media mentions, and online reviews, allows businesses to identify patterns and trends in customer behavior. This allows for proactive problem-solving and improved customer service. For example, a telecommunications company can use big data to identify common customer complaints and address them proactively through system improvements or targeted customer support initiatives.
This reduces the number of customer service calls, freeing up resources and lowering operational costs.
- Manufacturing: Predictive maintenance reduces equipment downtime, minimizing production losses and repair costs.
- Retail: Optimized inventory management reduces storage costs and minimizes stockouts, leading to improved sales and customer satisfaction.
- Healthcare: Improved patient care through personalized treatment plans and efficient resource allocation in hospitals.
- Finance: Fraud detection and prevention through real-time analysis of transaction data, minimizing financial losses.
- Transportation: Optimized routing and scheduling for delivery fleets, reducing fuel consumption and improving delivery times.
Big Data and Innovation
Big data isn't just about collecting information; it's a powerful engine for driving innovation across industries. The sheer volume, velocity, and variety of data available today provide unprecedented opportunities to identify trends, understand customer behavior, and develop entirely new products and services that were previously unimaginable. This transformative power allows businesses to move beyond reactive strategies and embrace proactive, data-driven innovation.The ability to analyze massive datasets reveals hidden patterns and insights that can inform the development of innovative products and services.
By understanding customer preferences, needs, and behaviors at a granular level, businesses can tailor offerings to specific segments, creating personalized experiences and increasing customer satisfaction. This data-driven approach also allows for the efficient identification of unmet needs and market gaps, paving the way for the creation of entirely new product categories.
Big Data's Role in New Product Development
Companies are using big data to create innovative products and services in various ways. For instance, analyzing customer feedback, social media trends, and purchasing patterns allows businesses to pinpoint unmet needs and design products that directly address them. Predictive modeling, enabled by big data analytics, allows for the accurate forecasting of demand, optimizing production and minimizing waste. This data-driven approach to product development minimizes risk and maximizes the likelihood of market success.
Netflix, for example, uses big data to analyze viewing habits, recommending personalized content and driving the creation of original programming tailored to specific audience preferences. This has led to the creation of successful shows and movies that cater to niche audiences and generate substantial viewership.
Examples of Disruptive Innovation Fueled by Big Data
Several companies have leveraged big data to create truly disruptive innovations. Consider the impact of Spotify's recommendation engine, which uses big data to analyze user listening habits and suggest new music. This personalized experience has transformed how people discover and consume music, disrupting traditional radio and music retail models. Similarly, the success of Amazon's recommendation system, which anticipates customer needs based on past purchases and browsing behavior, has fundamentally altered the landscape of e-commerce.
This personalized shopping experience drives sales and increases customer loyalty. These examples demonstrate how big data can not only improve existing products and services but also create entirely new paradigms.
Big Data and a Culture of Innovation
Big data fosters a culture of innovation by creating a data-driven decision-making environment. Access to real-time insights and predictive analytics empowers organizations to make informed decisions, experiment with new ideas, and rapidly iterate on products and services. The ability to measure the impact of various initiatives with precise data allows for continuous improvement and a more agile approach to innovation.
By fostering a culture of experimentation and learning from data, organizations can unlock their creative potential and drive significant competitive advantage. This data-driven approach encourages a culture of continuous improvement, allowing companies to adapt quickly to changing market conditions and stay ahead of the competition. The availability of comprehensive data allows for objective evaluation of innovation initiatives, ensuring that resources are allocated efficiently and effectively.
Big Data and Risk Management
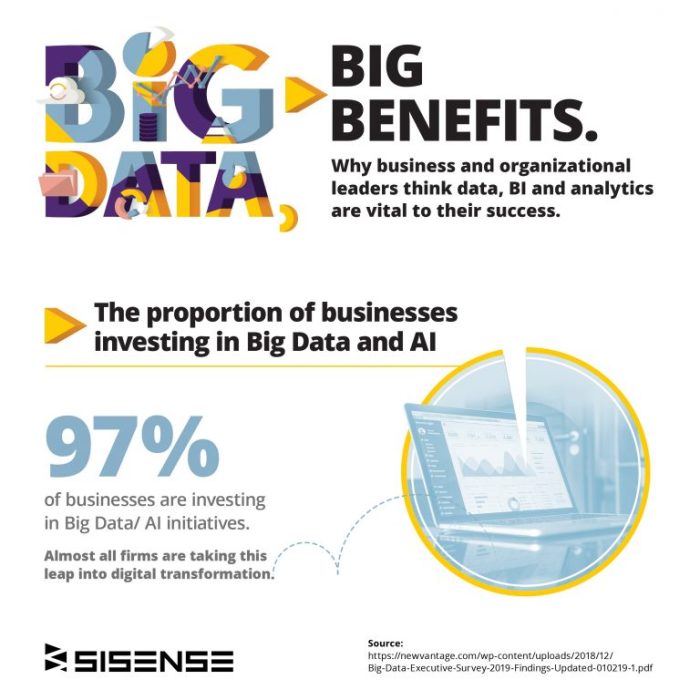
In today's interconnected world, organizations face a myriad of risks, from financial fraud to cybersecurity breaches. Big data analytics provides a powerful toolset for identifying, assessing, and mitigating these risks more effectively than ever before. By analyzing vast quantities of structured and unstructured data, businesses can gain unprecedented insights into potential threats and develop proactive strategies to minimize their impact.Big data's ability to process and correlate massive datasets allows for the identification of subtle patterns and anomalies that might otherwise go unnoticed.
This proactive approach to risk management shifts the focus from reactive damage control to predictive risk mitigation, leading to significant cost savings and improved operational resilience.
Fraud Detection and Prevention
Big data analytics plays a crucial role in detecting and preventing fraudulent activities across various sectors. Sophisticated algorithms can analyze transactional data, identifying unusual patterns indicative of fraudulent behavior. For instance, an algorithm might flag a series of unusually large or frequent transactions from a single account, a key indicator of potential credit card fraud. Similarly, analyzing customer behavior data can help identify suspicious login attempts or unusual purchasing patterns, potentially stemming from compromised accounts.
The speed and accuracy of big data analysis significantly improve the efficiency of fraud detection, allowing organizations to take immediate action and minimize losses. Real-time analysis enables the immediate blocking of suspicious transactions, preventing significant financial damage.
Big Data's Contribution to Enhanced Cybersecurity
Big data analytics strengthens cybersecurity defenses by providing a comprehensive view of network activity and potential threats. By analyzing massive logs of network traffic, security information and event management (SIEM) systems powered by big data can identify malicious activities in real-time. For example, an anomaly detection algorithm might flag a sudden surge in traffic from an unusual IP address, potentially indicating a distributed denial-of-service (DDoS) attack.
Furthermore, analyzing user behavior data can help identify insider threats, such as employees accessing sensitive data without authorization. The predictive capabilities of big data allow for the development of proactive security measures, such as strengthening network defenses or implementing stricter access controls, before a breach occurs. A real-world example is the use of big data analytics by financial institutions to detect and prevent sophisticated phishing attacks by identifying patterns in email communications and user login attempts.
Big Data and Personalized Experiences
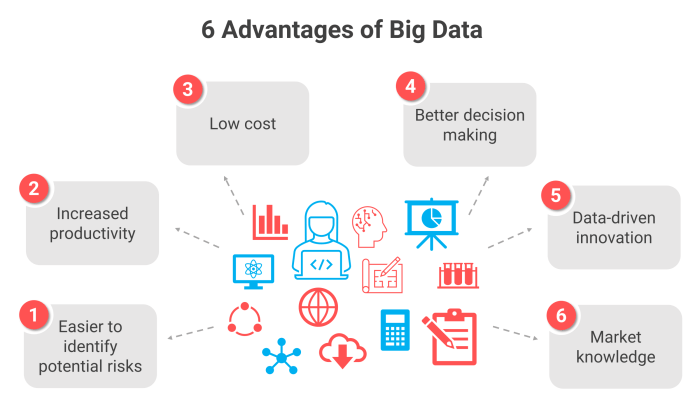
In today's hyper-competitive market, understanding and catering to individual customer needs is paramount. Big data, with its vast repositories of information, provides the key to unlocking truly personalized experiences, transforming how businesses interact with their clientele and fostering stronger, more profitable relationships. This allows companies to move beyond generic marketing strategies and deliver tailored interactions that resonate deeply with each customer.Big data enables personalized customer experiences by providing a granular understanding of individual customer behavior, preferences, and needs.
Through the analysis of vast datasets encompassing demographics, purchase history, website activity, social media engagement, and more, businesses can create detailed customer profiles. These profiles serve as the foundation for highly targeted marketing campaigns, product recommendations, and customer service interactions, resulting in increased customer satisfaction and loyalty.
Personalized Marketing Approaches Using Big Data
Several approaches leverage big data to personalize marketing efforts. One common method involves segmenting customers based on shared characteristics and behaviors. For example, a clothing retailer might segment its customer base by age, gender, style preferences, and past purchases to create targeted advertising campaigns featuring specific product lines. Another approach uses predictive modeling to anticipate future customer behavior.
By analyzing past purchase patterns and browsing history, businesses can predict what products a customer is likely to buy next, enabling proactive recommendations and offers. A third approach focuses on real-time personalization, adapting the customer experience in real-time based on their current actions and context. For instance, a website might display personalized product recommendations based on items currently in a customer's online shopping cart.
These approaches, while distinct, often complement each other to create a holistic and highly personalized marketing strategy.
Data-Informed Personalized Recommendations
Imagine a visual representation: a central node representing a single customer. From this node, numerous lines extend outward, each representing a data point. These lines connect to larger clusters representing various data sources: purchase history (showing specific items and dates), website activity (pages visited, time spent, items viewed), social media interactions (likes, shares, comments related to the brand), and demographic information (age, location, income).
The thickness of each line corresponds to the significance of that data point in shaping the customer's profile. Algorithms process this data to identify patterns and predict future behavior. Based on these predictions, personalized recommendations – represented as smaller nodes branching off from the main node – are generated, showcasing products or services tailored to the individual's unique preferences and needs.
This visual illustrates how diverse data points coalesce to inform highly targeted recommendations.
Challenges of Implementing Big Data
Harnessing the power of big data isn't without its hurdles. The journey from raw data to actionable insights requires careful planning, significant investment, and a proactive approach to potential pitfalls. Overcoming these challenges is crucial for organizations aiming to successfully leverage big data for competitive advantage.The implementation of big data solutions presents a multifaceted challenge, encompassing technical, organizational, and ethical considerations.
These challenges can significantly impact the success or failure of a big data initiative, making a thorough understanding of these obstacles essential for strategic planning and effective execution.
Data Security and Privacy
Protecting sensitive data is paramount in any big data initiative. The sheer volume and variety of data collected necessitate robust security measures to prevent breaches and maintain compliance with regulations like GDPR and CCPA. This includes implementing encryption, access control mechanisms, and regular security audits. Failure to adequately address data security can lead to significant financial losses, reputational damage, and legal repercussions.
For example, a data breach exposing customer personal information could result in hefty fines and a loss of consumer trust, severely impacting the company's bottom line. A multi-layered approach, incorporating both technical safeguards and strong organizational policies, is essential for maintaining data integrity and user privacy.
The Need for Skilled Professionals
Big data projects require a specialized skillset that combines technical expertise with business acumen. The demand for data scientists, data engineers, and big data architects far exceeds the current supply, creating a significant talent gap. Organizations struggle to find and retain professionals with the necessary skills to design, implement, and manage complex big data systems. This shortage can lead to project delays, increased costs, and a suboptimal utilization of big data resources.
Investing in training and development programs, as well as offering competitive compensation packages, are crucial strategies for attracting and retaining top talent in this competitive field. Furthermore, fostering a collaborative environment that encourages knowledge sharing and continuous learning can help organizations build a robust and skilled big data team.
Data Integration and Management
Integrating data from disparate sources presents a significant challenge. Data often resides in various formats and locations, making it difficult to consolidate and analyze. Inconsistencies in data quality and the presence of missing or inaccurate data further complicate the process. Effective data integration requires careful planning, the use of appropriate ETL (Extract, Transform, Load) tools, and robust data governance policies.
Without a well-defined data integration strategy, organizations risk creating data silos and hindering their ability to derive meaningful insights from their data. The cost and complexity of data integration can also be substantial, requiring significant investment in both technology and personnel. This complexity is further compounded by the need to ensure data consistency and accuracy throughout the integration process.
Scalability and Cost
Big data solutions require significant investment in infrastructure and technology. The cost of storing, processing, and analyzing large volumes of data can be substantial, particularly for organizations with limited resources. Furthermore, scaling big data systems to accommodate growing data volumes and increasing user demands can be complex and expensive. Careful planning and the selection of cost-effective technologies are essential for managing these costs.
A phased approach to implementation, starting with a pilot project and gradually scaling up as needed, can help organizations manage costs and mitigate risks. Choosing cloud-based solutions can also offer greater scalability and cost-efficiency compared to on-premises deployments. Careful monitoring of resource utilization and optimization strategies are vital for maintaining cost-effectiveness throughout the lifecycle of the big data project.
The Future of Big Data
The relentless growth of data, fueled by the proliferation of connected devices and the increasing digitization of every aspect of life, ensures that big data will remain a dominant force shaping our world. The future isn't just about handling more data; it's about harnessing its power with unprecedented sophistication and efficiency, driven by advancements in related technologies and a deeper understanding of its potential.
We stand at the cusp of a new era where big data transcends its current applications and becomes an integral part of virtually every industry and aspect of human endeavor.The next decade will witness a dramatic transformation in how we interact with and utilize big data. This evolution will be significantly influenced by the convergence of several powerful technologies, leading to more intelligent, adaptable, and insightful applications.
Predictive Analytics and AI-Driven Insights
The marriage of big data and artificial intelligence will yield unparalleled predictive capabilities. AI algorithms, trained on massive datasets, will move beyond simple pattern recognition to anticipate future trends with greater accuracy. For example, in healthcare, AI could analyze patient data to predict disease outbreaks or personalize treatment plans with a precision currently unimaginable. In finance, AI-powered systems will refine risk assessment models, leading to more efficient investment strategies and fraud detection.
The increased sophistication of these predictive models will lead to proactive decision-making across various sectors, improving efficiency and mitigating potential risks.
The Expanding Role of the Internet of Things (IoT)
The IoT's explosive growth is generating an unprecedented flood of data from connected devices. This data, integrated with existing big data repositories, will create a rich, real-time understanding of the physical world. Imagine smart cities leveraging IoT data to optimize traffic flow, manage energy consumption, and enhance public safety. In manufacturing, IoT sensors could monitor equipment performance, predict maintenance needs, and minimize downtime.
This seamless integration of data from the physical and digital realms will unlock new levels of automation and efficiency across numerous industries.
Big Data's Continued Transformation of Industries
Big data's influence will continue to deepen across various sectors. In retail, personalized recommendations and targeted advertising will become even more refined, leading to enhanced customer experiences and increased sales. In transportation, big data will optimize logistics, improve traffic management, and contribute to the development of autonomous vehicles. The media and entertainment industries will leverage big data to create more engaging content, tailor experiences to individual preferences, and improve content distribution strategies.
Essentially, big data will become the lifeblood of innovation and efficiency across the board. For example, Netflix's success in personalized content recommendations is a testament to the power of big data in shaping consumer experiences and driving business growth.
Enhanced Data Security and Privacy Measures
As the volume and sensitivity of big data increase, so too will the need for robust security and privacy measures. The future of big data will involve the development of sophisticated encryption techniques, advanced threat detection systems, and stricter regulatory frameworks to protect sensitive information. This focus on data security will be crucial in maintaining public trust and ensuring the responsible use of big data technologies.
The implementation of blockchain technology, for instance, offers potential solutions for secure data management and transparent data sharing.
The potential of big data is undeniable. Its ability to transform businesses, personalize experiences, and drive innovation is already reshaping our world. While challenges remain in terms of data security, privacy, and the need for skilled professionals, the rewards far outweigh the risks. As technology continues to evolve, particularly with the integration of artificial intelligence and the Internet of Things, big data's influence will only grow stronger.
Embracing this technology and leveraging its power strategically is no longer a luxury; it's a necessity for businesses seeking to thrive in the increasingly data-driven landscape of the future. The insights gained from big data analysis translate directly into competitive advantages, improved efficiency, and ultimately, sustained success.
FAQ
What are the ethical concerns surrounding big data?
Ethical concerns revolve around data privacy, bias in algorithms, and the potential for misuse of personal information. Responsible data handling, robust security measures, and transparent data practices are crucial to mitigate these risks.
How much does it cost to implement a big data solution?
The cost varies greatly depending on the scale of the project, the required infrastructure, and the expertise needed. Smaller businesses might start with cloud-based solutions, while larger enterprises may require significant investments in hardware and personnel.
What types of jobs are available in the big data field?
The field offers diverse roles, including data scientists, data engineers, data analysts, database administrators, and big data architects, each requiring different skill sets and experience levels.
How can small businesses benefit from big data?
Small businesses can leverage affordable cloud-based analytics tools to gain insights into customer behavior, optimize marketing campaigns, and improve operational efficiency, even on a limited budget.
What is the difference between big data and business intelligence?
While both involve data analysis, big data focuses on analyzing massive, complex datasets often using advanced techniques, whereas business intelligence typically deals with smaller, structured data using more traditional methods.